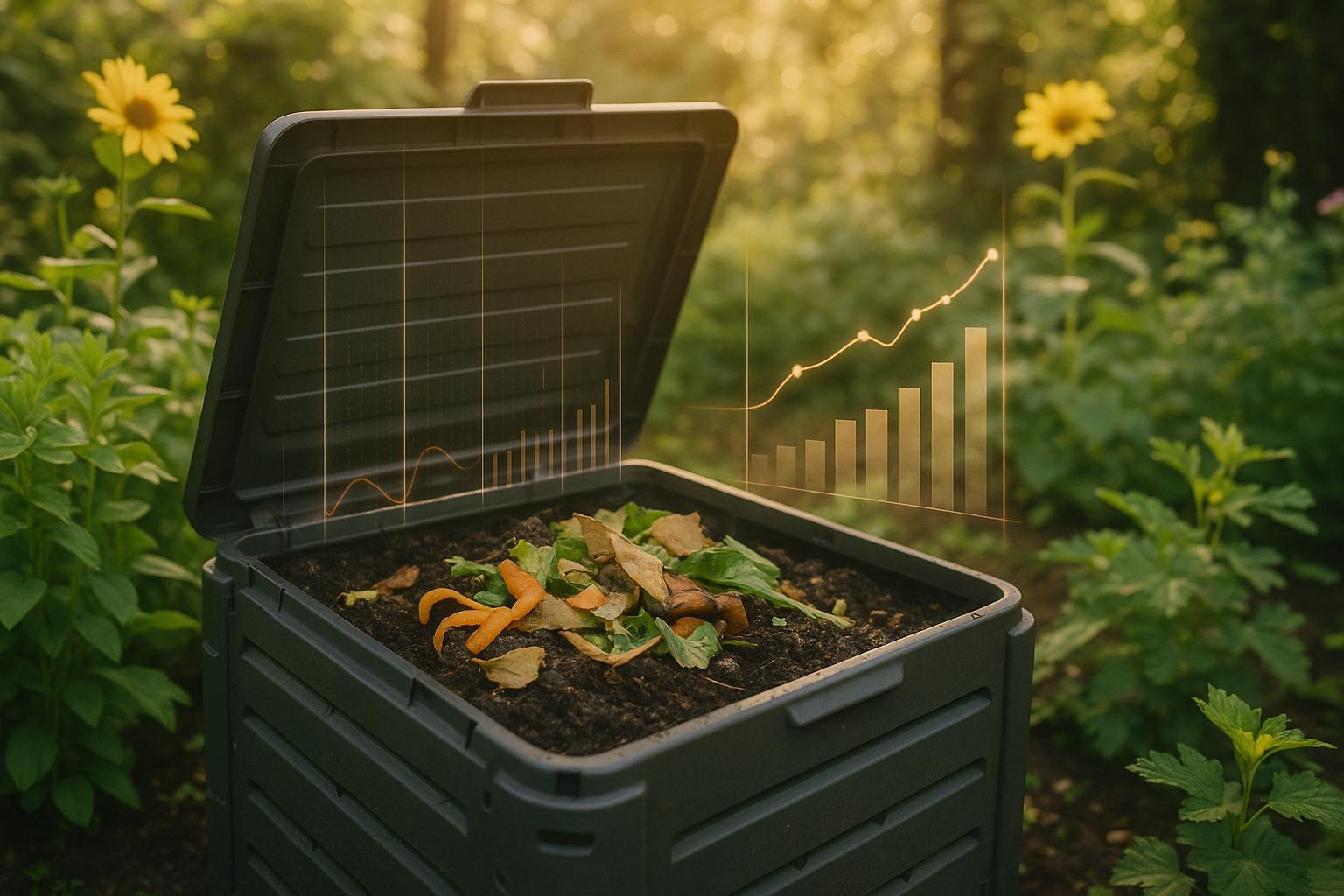
Explore how AI models are revolutionizing composting by enhancing efficiency, reducing costs, and improving compost quality through real-time monitoring and predictions.
AI Models for Compost Decomposition Rates
AI is transforming composting by predicting decomposition rates, improving efficiency, and reducing costs. With tools like neural networks and IoT sensors, facilities can now monitor and optimize composting in real time. Here’s what AI brings to the table:
- Accurate Predictions: Models achieve R² scores up to 0.99 for key metrics like carbon-to-nitrogen ratios and compost maturity.
- Real-Time Monitoring: AI tracks temperature, moisture, pH, gas emissions, and microbial activity for better process control.
- Faster Decomposition: AI-powered systems speed up composting by up to 30% and reduce greenhouse gas emissions by 40–60%.
- Cost Savings: Municipal facilities report 30% lower operational costs and 25% less material loss using AI systems.
- Better Compost Quality: AI fine-tunes nitrogen and carbon levels, ensuring higher-quality compost for farming.
Leveraging AI and Machine Learning for Soil Health
Current AI Models for Composting
Today's AI technologies in composting utilize advanced neural networks paired with real-time sensor data to better predict decomposition rates. These models often achieve R² values between 0.70 and 0.99, showcasing their reliability in practical applications.
Neural Networks for Time-Based Analysis
Modern composting operations increasingly depend on Long Short-Term Memory (LSTM) networks and other advanced neural architectures to process environmental data. These systems are particularly adept at identifying time-based patterns in composting parameters, offering clear advantages over traditional linear models.
Physics-informed neural networks (PINNs) are another major step forward in composting analysis. By integrating fundamental conservation equations, these models deliver highly accurate and physically consistent predictions of decomposition rates. This capability allows facility operators to maintain ideal conditions throughout the composting process.
For example, an artificial neural network (ANN) monitoring the composting of sewage sludge mixed with straw achieved an impressive R² score above 0.97 by analyzing factors like:
- Temperature
- pH levels
- Electrical conductivity
- Carbon-to-nitrogen ratio
- Ammonium nitrogen concentration
These advancements underscore how neural networks are reshaping the composting landscape, particularly in microbiological monitoring.
AI Analysis of Microbial Activity
AI doesn't just excel at analyzing time-based data - it also plays a pivotal role in monitoring biological factors. By tracking microbial populations with remarkable precision, AI systems have significantly improved decomposition efficiency. These models can predict bioremediation outcomes and fine-tune microbial consortia in real time.
One study highlighted how an ANN model accelerated fluoranthene degradation. The AI-optimized approach achieved 51.28% degradation in just three days, compared to only 26.37% after seven days using traditional methods:
Timeframe | AI-Optimized Degradation | Traditional Approach |
---|---|---|
3 days | 51.28% degradation | - |
7 days | - | 26.37% degradation |
AI systems dynamically adjust key parameters, such as microbial inoculation, nutrient levels, and oxygen supply. This adaptability has enabled facilities to reduce cumulative greenhouse gas emissions by 40–60% during their composting cycles.
These models also excel at predicting compost maturity and quality indicators, including enzymatic activity, moisture levels, carbon-to-nitrogen ratios, and greenhouse gas outputs.
Multi-Sensor Systems and AI Integration
Modern composting facilities are stepping up their game by using integrated multi-sensor networks to monitor decomposition in real time. These systems are transforming how composting conditions are tracked and managed.
IoT and Imaging Sensors
By combining traditional sensors with imaging technology, these advanced systems create a detailed picture of the composting process. They monitor essential factors like temperature, moisture, and oxygen levels, while also using computer vision to analyze material structure and spot contaminants.
Here’s what this integration brings to the table:
- 40% reduction in manual soil sampling
- Real-time detection of contamination
- Tracking material density and structural changes
These innovations have also enabled faster, on-site data analysis, thanks to the use of edge computing technology.
Edge Computing Applications
Edge computing has taken composting efficiency to a new level. For instance, the COMPaaS system has shown it can speed up composting by as much as 30% compared to traditional methods.
Parameter | Improvement |
---|---|
Energy Consumption | 25% reduction with IoT-integrated systems |
Processing Time | 30% faster decomposition rates |
Response Time | Near-instant adjustments |
Data Processing | Local handling of routine tasks |
With edge computing, sensor data is processed directly on-site, allowing for immediate adjustments to critical parameters like aeration and moisture. This means composting machinery equipped with sensors and actuators can handle routine tasks locally.
For large-scale facilities, this level of precision is a game changer. Automated adjustments to aeration, moisture levels, and turning schedules ensure consistent decomposition across massive batches, significantly improving overall efficiency and quality.
sbb-itb-4d6a8dd
🚀 Ready to Reinvent Your Garden?
Join thousands of homeowners who have transformed their gardens using our AI design tool. Upload one photo to explore endless possibilities.
Get your AI garden designs →AI Model Results in Practice
AI models are transforming composting operations by improving efficiency, enhancing quality, and cutting costs across various scales.
City Waste Processing Results
Municipal facilities adopting AI-driven analytics have seen impressive results, including a 25% reduction in material loss and a 40% boost in energy efficiency. These improvements are particularly evident in waste sorting and processing operations:
Improvement Area | Results |
---|---|
Contamination Reduction | Over 60% decrease with AI-powered sorting |
Operational Costs | 30% lower costs with IoT technology |
Processing Time | 20–30% faster without compromising quality |
Equipment Maintenance | 50% fewer breakdowns |
As municipal waste volumes continue to grow, these efficiencies have become increasingly vital. AI-powered monitoring systems play a key role by continuously tracking critical parameters and providing real-time feedback for optimizing processes. While cities gain from these advancements, farms are also reaping the rewards of AI integration.
Farm Waste Processing Results
In agricultural composting, AI technology is revolutionizing resource management with measurable outcomes. Smart systems have replaced traditional methods, offering precise and efficient solutions:
Application | Impact |
---|---|
Digital Soil Mapping | 40% fewer physical samples required |
Crop Yield Prediction | 25% more accurate forecasts |
Nitrogen Fertilizer Use | 15% reduction in costs |
Water Management | 30% less water usage |
These advancements are powered by real-time sensors and edge computing, which allow for dynamic adjustments to maintain ideal conditions. Energy-efficient systems have cut energy consumption by 25%, while AI-controlled parameters ensure consistent composting conditions. Machine learning algorithms analyze a variety of factors - such as composting parameters, environmental conditions, and feedstock characteristics - to predict outcomes with high accuracy. In urban farming, AI systems even anticipate temperature shifts based on weather forecasts, enabling proactive adjustments to maintain optimal conditions.
Limitations and Future Development
While AI shows promise in compost management, several challenges hinder its broader adoption and effectiveness.
Data Quality Issues
The success of AI in composting heavily depends on the quality and consistency of the data it processes. However, several hurdles remain:
Challenge | Impact | Potential Solution |
---|---|---|
Inconsistent Data Collection | Reduces model accuracy across facilities | Standardized collection protocols |
Limited Training Datasets | Weakens the model's ability to generalize | Facility-wide data sharing |
Feedstock Diversity | Makes standardizing predictions difficult | Expanded data collection for diverse waste types |
The dynamic nature of composting, including varying environmental conditions and waste compositions, adds complexity to data standardization.
"AI-driven compost quality prediction and optimization signifies a paradigm shift in sustainable waste management, enhancing efficiency and environmental benefits." - Sustainability Directory
To overcome these obstacles, researchers are exploring advanced AI techniques designed to handle these complexities.
Advanced AI Methods
Emerging AI technologies are being developed to tackle the limitations of current systems and improve the reliability and efficiency of compost management:
AI Method | Application | Expected Impact |
---|---|---|
Reinforcement Learning | Real-time process optimization | Enables continuous adjustments to parameters |
Generative Models | Dataset augmentation | Enhances model training and generalization |
Explainable AI (XAI) | Process transparency | Provides clearer insights into quality factors |
Edge Computing | Local data processing | Reduces latency and improves response times |
However, there are risks and barriers to consider. Over-reliance on AI could lead to a decline in operators' practical knowledge. Additionally, rural areas face unique challenges, such as limited internet connectivity and hardware durability issues. To address these gaps, localized solutions are essential.
Collaboration between academic institutions and industry partners is crucial to overcoming these challenges. By working together, they can improve data quality, enhance AI interpretability, and ensure practical applications meet real-world needs.
Conclusion: AI's Impact on Composting
AI technology is reshaping how compost is managed, bringing in tools like predictive analytics and real-time controls to streamline the process. Machine learning algorithms, such as Random Forest and Extra Tree methods, have shown impressive accuracy in predicting decomposition metrics, with R² values of about 0.937 and 0.904, respectively, for the germination index.
In San Antonio, Texas, AI-powered robotic sorting systems have made a big difference by eliminating organic waste rejections due to contamination - a common issue with older methods. These systems also fine-tune moisture and oxygen levels, cutting ammonia emissions by 30%. On top of that, logistics improvements have delivered measurable results:
Improvement Area | Impact |
---|---|
Transportation Distance | 36.8% reduction |
Operational Costs | 13.35% savings |
Processing Time | 28.22% improvement |
Waste Sorting Accuracy | Up to 99.95% |
Sustainability Directory: "AI is poised to become an indispensable tool in the quest for a more circular and environmentally responsible future, starting with the humble yet vital process of composting."
These AI-driven systems not only improve logistics but also produce higher-quality compost while reducing greenhouse gas emissions through optimized processing. This shift marks a move from reactive waste management to proactive and efficient solutions in sustainable practices.
The combined benefits of AI - greater efficiency, better compost quality, and reduced environmental impact - highlight its essential role in modern waste management. As more municipalities and facilities adopt these technologies, advancements in AI models are focusing on handling diverse feedstock types and adapting to varying environmental conditions. These developments hold the promise of further improving composting processes, minimizing ecological footprints, and enhancing compost quality for agricultural use.
FAQs
How are AI models making composting faster, cheaper, and more sustainable?
AI is reshaping the composting process by fine-tuning critical elements like temperature, moisture levels, and airflow - factors that are key to effective decomposition. Using real-time data, these systems can predict when compost will be ready and make adjustments to optimize conditions. This approach not only accelerates the process but also helps cut down on operating expenses.
On top of that, AI-driven tools take over tasks like monitoring and management, reducing the reliance on manual labor. They also play a role in lowering emissions generated during composting, aligning with regulatory requirements and promoting eco-friendly practices. The result? A more efficient, cost-effective, and environmentally conscious way to handle organic waste.
How do neural networks and IoT sensors improve real-time compost monitoring?
Neural networks and IoT sensors are transforming real-time compost monitoring by automating how decomposition conditions are tracked and optimized. IoT sensors collect essential data, such as temperature, moisture, and oxygen levels, all of which are crucial for creating the perfect environment for composting. This information is then processed by neural networks, which analyze the data to predict decomposition rates and suggest adjustments to keep the process on track.
By combining these technologies, composting becomes faster and produces higher-quality results. This approach not only maximizes efficiency but also helps gardeners and compost managers manage resources more effectively while improving overall sustainability.
What challenges does AI face in managing compost decomposition, and how can they be solved?
AI in compost management comes with its fair share of challenges. High setup costs, the need for trained operators, and the struggle to integrate AI into existing composting systems are some of the significant hurdles. On top of that, the natural complexity of composting - shaped by variables like temperature, moisture levels, and the composition of materials - makes accurate predictions a tough nut to crack.
Addressing these challenges requires developing AI tools that are both cost-effective and easy to use, ensuring they can blend seamlessly with the systems already in place. Offering proper training to operators is another key step, as it can make the adoption process smoother and lead to better outcomes. Ongoing research aimed at fine-tuning composting variables with the help of AI will also play a big role in improving prediction accuracy and boosting the quality of the compost produced.
🎨 Visualize Your Dream Garden Today!
Transform any outdoor space into a professional landscape design in minutes. Just upload a photo, choose your style, and let our AI do the rest.
Start your garden transformation now →