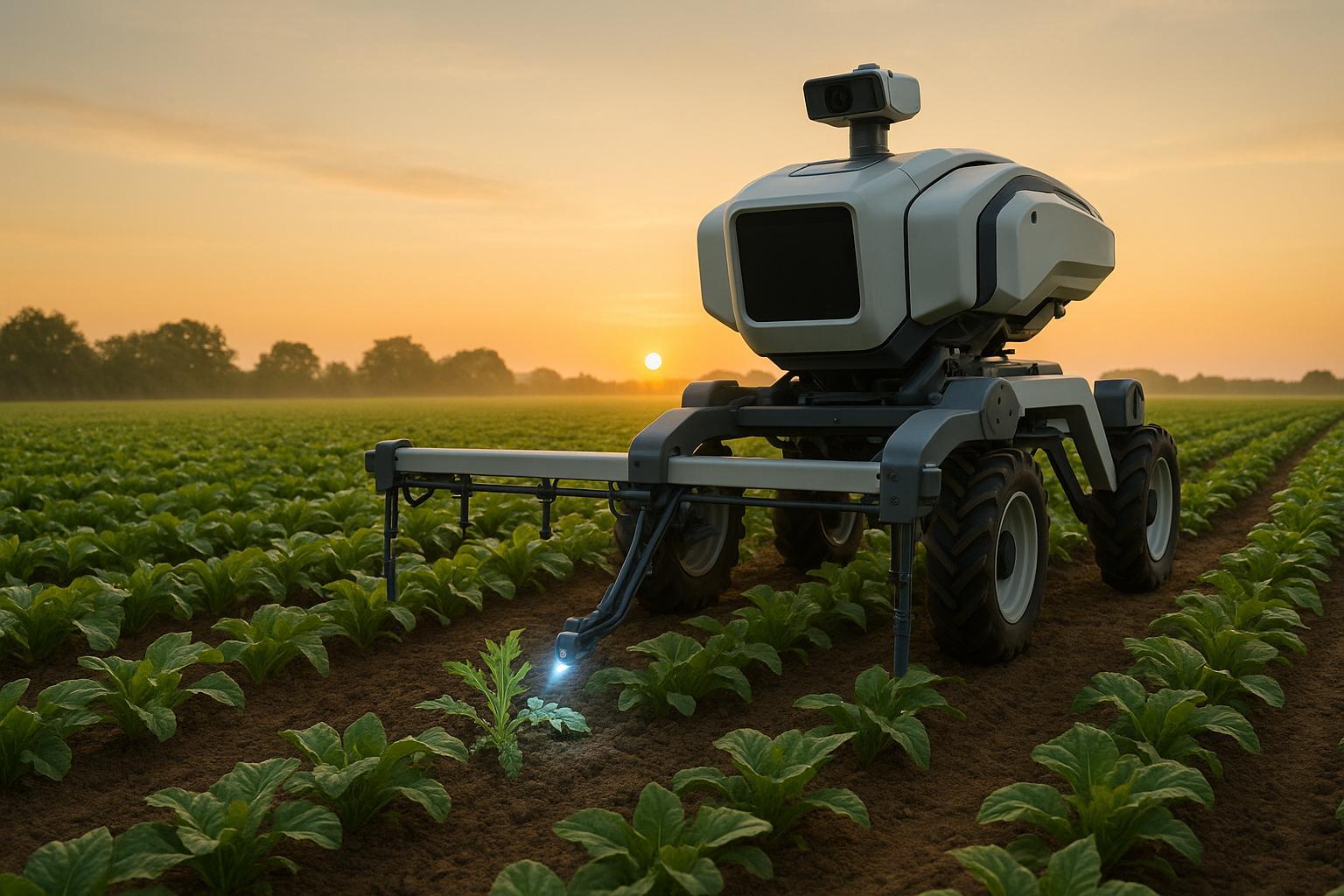
Explore how user-friendly design principles in AI weed control systems enhance efficiency, reduce costs, and transform modern agriculture.
Designing Interfaces for AI Weed Control Systems
AI weed control systems are changing farming by reducing herbicide use by up to 90% and cutting weeding costs by over 50%. These tools combine machine learning, computer vision, and robotics to identify and remove weeds with precision, saving farmers time and money while minimizing chemical use.
Key Takeaways:
- Labor Savings: Reduces manual labor by up to 40.5 hours per acre.
- Cost Efficiency: Cuts costs by $15β25 per acre through reduced herbicide use.
- Precision: Systems like John Deereβs See & Spray achieve up to 90% herbicide reduction.
- User-Friendly Features: Real-time visuals, offline functionality, and intuitive controls make the technology accessible to farmers.
Why Interface Design Matters:
- Improves Adoption: 60% of agricultural tech remains unused due to poor usability.
- Boosts Productivity: Tailored interfaces improve yield management for 72% of users.
- Handles Challenges: Designed for offline use and harsh field conditions.
With the agricultural AI market projected to grow to $4.7 billion by 2028, designing simple, effective interfaces is critical for making this technology practical for everyday farming.
Design Principles for AI Weed Control Interfaces
Simple Yet Powerful Design
When crafting interfaces for AI weed control systems, the goal is to strike a balance between ease of use and robust functionality. The design should avoid overwhelming users with technical jargon while still delivering precise botanical details that cater to both novices and experts alike.
Transform Your Garden Today - First Design FREE!
Turn your outdoor space into a stunning landscape. No credit card required.
Key elements of effective interfaces include:
- Camera positioning guidance: Clear visual cues to help users capture the best images.
- Prioritized data layout: Presenting the most critical information first, followed by supplementary details.
- Location-aware functionality: Incorporating GPS to provide climate-specific advice.
"Intuitive navigation makes the difference between frustration and functionality in weed identification apps."
Making AI Actions Clear
Transparency is essential for building trust in AI systems and ensuring users can operate them effectively. Google's approach to AI transparency provides a great example, with features like "Why this result?" offering clear explanations for search suggestions.
π¨ Transform Your Garden Today
FREETurn your outdoor space into a stunning landscape
Join 10,000+ happy gardeners β’ No credit card needed
Transparency Element | Implementation Strategy | User Benefit |
---|---|---|
Visual Indicators | Confidence levels and progress bars | Helps users understand AI certainty |
Context Clues | Explanations like "Based on your field conditions" | Offers insight into AI decisions |
Error Correction | Suggestions like "Did you mean this?" | Allows quick fixes for misidentifications |
User Override Options | Manual adjustments | Gives users control over AI actions |
Meeting Different User Skill Levels
To engage users of all expertise levels, interfaces must offer flexibility while promoting ease of learning. Progressive disclosure - introducing features step by step - helps users get comfortable with the system without feeling overwhelmed.
Key features for accommodating diverse skill sets include:
- Multi-layer displays and flexible inputs: Support for touch, voice, and gesture controls to meet varying user needs.
- Customizable control strategies: Options for managing both chemical and non-chemical weed treatments.
- Offline functionality: Ensuring usability in areas with limited or no connectivity.
Visual aids like confidence meters and decision trees can simplify AI logic for users with varying technical knowledge. For instance, Google's People + AI Research (PAIR) initiative employs interactive dashboards, heatmaps, and decision trees to make AI decisions more accessible to non-technical users.
"We need transparency and ethical design choices to create experiences that clearly show how algorithms work, how systems handle data, and how AIs make decisions - all while addressing concerns such as users' skepticism, fear of automation, and privacy needs." - Magnus Eriksen
These principles lay the groundwork for thorough interface testing, paving the way for on-site assessments and user feedback to refine the design further.
Must-Have Features for Weed Control Interfaces
Live Weed Detection Display
A live weed detection display is essential for visualizing weeds in real time. By overlaying bounding boxes on the source image, users receive instant feedback on weed presence, achieving accuracy rates of over 90%, even in challenging lighting conditions.
Here are some key components of the display:
Feature | Function | User Benefit |
---|---|---|
Detection Counter | Shows the number of weeds in the frame | Provides a quick overview of infestation levels |
Confidence Meter | Displays the AI's certainty percentage | Helps users gauge detection reliability |
Geographic Filter | Utilizes location data to refine results | Improves precision in weed identification |
Camera Controls | Adjusts exposure, gain, and gamma settings | Enhances image quality for better detection |
These tools ensure users have clear, actionable insights, setting the foundation for effective field operations.
Field Operation Controls
Outdoor environments can pose challenges to control panel functionality. The National Electrical Manufacturers Association (NEMA) emphasizes the importance of selecting appropriate enclosures to shield electronic components.
To ensure durability and performance, control panels should operate in conditions below 95Β°F with relative humidity under 60%. Depending on the environment, these enclosure types are recommended:
Environment Type | Recommended Enclosure | Key Features |
---|---|---|
Standard Outdoor | Type 4 | Weather-resistant; protects against water spray |
Dusty Areas | Type 5 | Dust-tight seals to prevent contamination |
Wet Conditions | Type 6 | Waterproof, even for submersion up to 6 ft |
Chemical Exposure | Type 13 | Resistant to oil and coolant sprays |
These enclosures ensure that field controls remain functional, even in harsh conditions. Pairing this durability with user feedback enhances system performance further.
User Feedback Systems
User feedback plays a pivotal role in refining weed control interfaces. Google's People + AI Research team highlights this importance:
"For AI products, user feedback and control are critical to improving your underlying AI model's output and user experience. When users have the opportunity to offer feedback, they can play a direct role in personalizing their experiences and maximizing the benefit your product brings to them. When users have the right level of control over the system, they're more likely to trust it."
An effective feedback system should incorporate the following features:
- Visual Indicators: Real-time displays of detection confidence levels.
- Haptic Feedback: Vibration alerts to notify users of detection events.
- Audio Cues: Sound notifications to communicate system status.
- Performance Metrics: Continuous tracking of detection accuracy for improvement.
By analyzing user input through machine learning algorithms, the system can identify patterns and adapt to improve accuracy.
As one expert notes:
"Weed identification apps are revolutionizing home gardening and professional agriculture alike by making expert plant knowledge accessible to everyone regardless of their botanical expertise."
These features create an interface that not only adapts to user needs but also maintains high accuracy across a variety of field conditions. Regular updates based on user feedback ensure the system remains effective and user-friendly.
Smart Cultivator & Weeder By Stout Industrial Technology
sbb-itb-4d6a8dd
π Ready to Reinvent Your Garden?
Join thousands of homeowners who have transformed their gardens using our AI design tool. Upload one photo to explore endless possibilities.
Interface Testing Methods
Thorough testing methods are key to ensuring the functionality and usability of AI weed control interfaces. Here's how different approaches contribute to refining these systems.
On-Site Testing
Testing in real-world conditions is indispensable for evaluating how AI weed control interfaces perform outside the lab. Field trials uncover issues that controlled environments might overlook, offering a clearer picture of operational efficiency and potential environmental impacts. Validation trials conducted with external centers further assess ecotoxicology and overall performance. After these trials, evaluating how much user effort is required can provide additional insights into how intuitive and user-friendly the interface truly is.
User Effort Assessment
Understanding how users interact with the interface is critical. Metrics like task completion times, navigation ease, and learning curves are all evaluated to measure usability. Tools such as Axe and WAVE help automate code compliance checks, while screen reader testing ensures accessibility for all users.
"Testsigma has been an absolute game-changer for us. We've saved time and caught critical issues that would've been missed with manual testing."
β Bharathi K
These assessments help identify areas for improvement, ensuring that the interface is not only functional but also accessible and efficient for end users.
Weather and Wear Testing
Environmental testing ensures that the interface can withstand the challenges posed by outdoor agricultural environments. These tests simulate various weather conditions, such as temperature swings, high humidity, and prolonged exposure to sunlight, to verify the interface's durability. By exposing components to extreme conditions, manufacturers can ensure the interface remains reliable over time.
"Environmental testing confirms the reliability and longevity of products, components, and structures across diverse industries. It's where designs are subjected to the rigors of real-world conditions, ensuring they can withstand extreme temperatures, humidity, vibrations, and a host of other environmental stressors."
Borrowing techniques from civil engineering, these tests reinforce the importance of durability and reliability in agricultural interface design, ensuring the technology can handle the demands of real-world use.
Conclusion
By combining thoughtful design principles with user feedback, AI weed control systems are delivering measurable improvements in agriculture.
Main Points
AI-powered weed control tools are transforming farming practices by significantly reducing herbicide use and labor demands. Research indicates these systems can cut herbicide usage by 60β70% while saving farmers $15β25 per acre. Additionally, they help users reclaim 5β7 hours of work per week.
Modern interfaces strike a balance between advanced capabilities and ease of use, catering to users with varying skill levels. Features like live weed detection displays and responsive feedback mechanisms directly enhance efficiency. Meanwhile, weather-resistant controls ensure consistent performance in tough field conditions.
"Fundamentally, we can employ deep learning methods to train the algorithm we're using to work for any crop. This will make the technology useful in all weed scenarios with which farmers are faced."
β Oliver Scholz, Deputy Head, Contactless Test and Measuring Systems, Fraunhofer IIS
These advancements lay the groundwork for even more sophisticated AI interfaces in the future.
Next Steps in AI Interfaces
The AI weed control industry is expected to grow to $4.7 billion by 2028. Building on proven design and testing methodologies, future development will focus on areas like edge processing, hybrid equipment integration, and AI-assisted decision-making tools.
The next wave of innovation aims to combine technological advancements with ethical, user-centered design principles.
"We need AI that listens, literally and metaphorically, because the real innovation isn't the algorithm. It's what happens when a farmer's voice shapes the science."
FAQs
How do AI weed control systems make their interfaces easy to use while offering advanced features for all users?
AI weed control systems strike a careful balance between being easy to use and offering advanced features. They focus on user-friendly design with intuitive layouts, straightforward instructions, and simple navigation. This makes it easy for beginners to get started without feeling lost or overwhelmed.
For those with more experience, the systems also offer customizable settings and detailed data insights, allowing them to unlock the full potential of the technology.
By following user-centered design principles, these systems evolve based on feedback, ensuring they remain accessible to everyone - from first-time users to seasoned experts. No matter your skill level, they combine simplicity with powerful tools to meet your needs.
How does interface design impact the usability and success of AI weed control systems in agriculture?
The Role of Interface Design in AI Weed Control Systems
Interface design plays a crucial role in making AI weed control systems practical and easy to use for farmers. By simplifying complex AI technologies, a well-thought-out interface ensures that even individuals without a technical background can operate these tools effortlessly. This ease of use not only boosts accessibility but also encourages more farmers to adopt these systems, helping them fully leverage the technology.
A user-friendly interface also streamlines efficiency by offering quick access to essential information and tools. This enables farmers to make smarter, faster decisions about weed management, which can lead to healthier crops and better farming practices. With intuitive layouts and straightforward navigation, interface design becomes a key driver in the success of AI-powered solutions for agriculture.
How do environmental and field conditions affect the performance and durability of AI weed control interfaces?
Environmental and field conditions significantly impact how well AI weed control systems perform. Extreme weather - like heavy rain, strong winds, or intense heat - can throw off sensor readings, causing inaccurate data or even system breakdowns. Additionally, factors like soil type and moisture levels can affect how effectively sensors and algorithms identify weeds.
Field-specific challenges, such as thick crop growth or weeds with different shapes and sizes, can make it harder for the system to operate smoothly. For these systems to work reliably, they need to be built tough enough to handle unpredictable agricultural conditions, including harsh weather and physical wear from daily use.
π¨ Visualize Your Dream Garden Today!
Transform any outdoor space into a professional landscape design in minutes. Just upload a photo, choose your style, and let our AI do the rest.