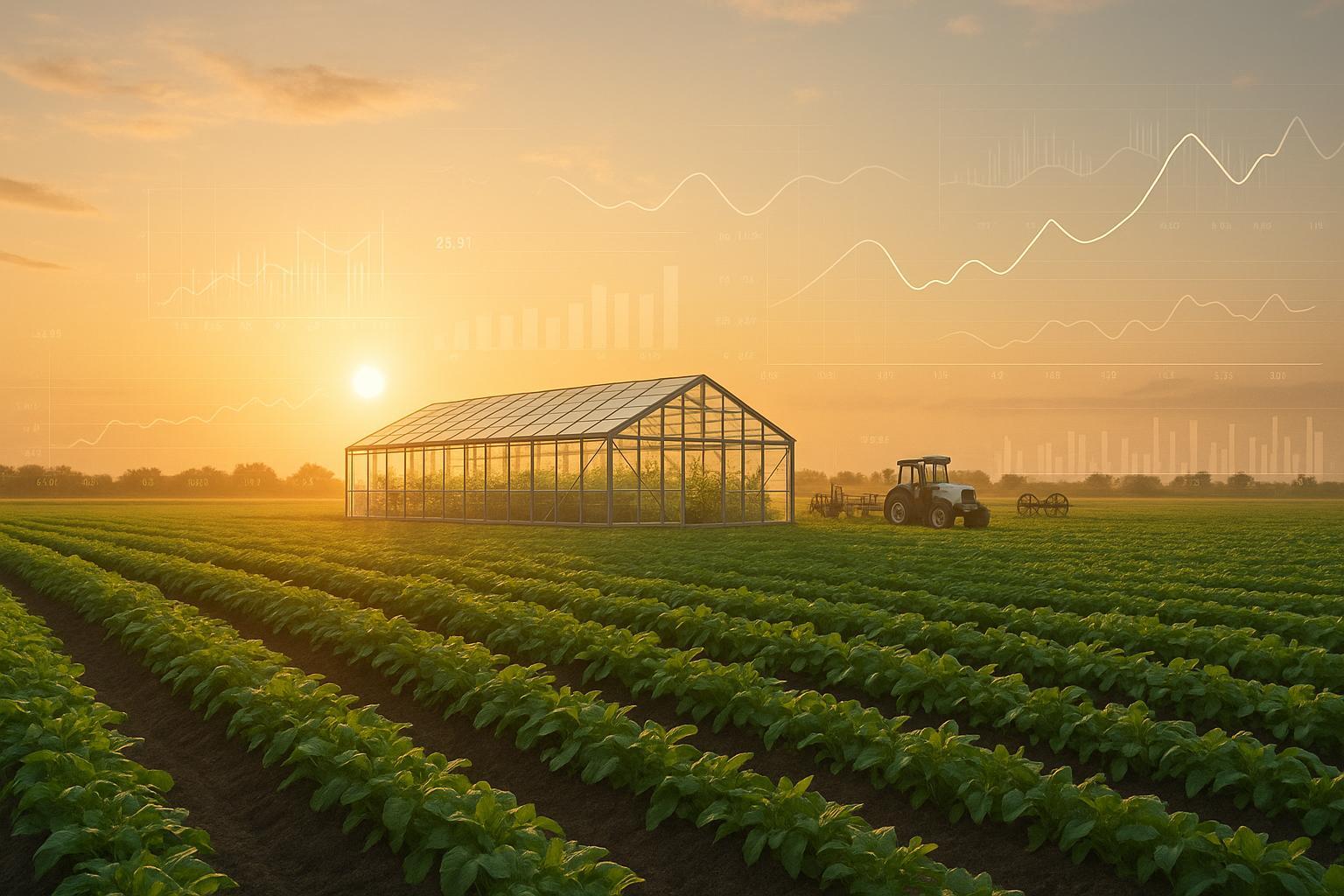
Explore how machine learning revolutionizes plant growth predictions, optimizing resources and improving yields for farmers and gardeners alike.
How Machine Learning Models Forecast Plant Growth
Machine Learning (ML) is transforming how we predict plant growth by analyzing data like weather, soil conditions, and crop yields. Here's what you need to know:
- Why It Matters: ML can reduce water use by 20–30%, cut fertilizer costs by 15–25%, and increase crop yields by 10–15%.
- Key Techniques: Algorithms like Random Forest (95.9% accuracy) and XGBoost (95.7% accuracy) are widely used. Neural networks handle complex environmental variables, while 3D imaging tracks plant changes in detail.
- Challenges: Limited data collection, inconsistent formats, and unpredictable weather hinder ML's full potential.
- New Advances: Virtual plant models, distributed learning, and AI-driven tools now optimize water, fertilizer, and planting strategies.
ML isn't just for large farms - it's powering tools for home gardeners too, helping with planting schedules, soil monitoring, and stress detection.
ML Method | Use Case | Accuracy |
---|---|---|
Random Forest | Yield prediction | 95.9% |
XGBoost | Resource optimization | 95.7% |
3D Imaging | Plant structure monitoring | 88.13% F1 |
ML is reshaping farming and gardening, making growth predictions smarter and more efficient.
ML Model Functions in Growth Prediction
Common ML Methods for Growth Analysis
Machine learning models use a variety of algorithms to study and predict plant growth patterns. For instance, research highlights that Random Forest achieved an impressive R² value of 0.958968 (about 95.9% accuracy), while XGBoost closely followed with an R² value of 0.956815 (approximately 95.7% accuracy). Artificial Neural Networks (ANNs) are often employed to predict crop yields by handling complex environmental variables. Deep Neural Networks (DNNs) take this further by utilizing multiple hidden layers for deeper analysis. Other methods include LSTM networks, which excel in time-series data analysis, and Convolutional Neural Networks (CNNs), often used for visual data interpretation.
Algorithm Type | Primary Application | Typical Accuracy |
---|---|---|
Random Forest | Overall yield prediction | 95.9% |
XGBoost | Resource optimization | 95.7% |
The success of these models hinges on diverse and precise data inputs.
Required Data Types
To create accurate predictions, ML models depend on a wide range of data inputs. A review of successful implementations reveals the most commonly utilized data categories:
Data Category | Frequency of Use |
---|---|
Temperature | 24 |
Soil Type | 17 |
Rainfall | 17 |
Humidity | 11 |
Solar Radiation | 10 |
Fertilization | 7 |
These inputs provide the foundation for reliable growth forecasts.
Prediction Process Steps
Once the necessary data is collected, ML models follow a structured workflow to generate predictions. Here’s a breakdown of the key steps:
-
Data Collection and Preprocessing
Environmental data - such as temperature, soil conditions, and rainfall - is gathered using sensors and field measurements. For instance, the Hot Serial Cereal Experiment tracked temperature ranges from 28°F (-2°C) to 107.6°F (42°C). -
Model Training and Validation
Historical datasets are used to establish baseline growth patterns, which help in forecasting planting schedules and yield outcomes. -
Performance Evaluation
Models are evaluated using standardized metrics to ensure accuracy. Common evaluation parameters include:Evaluation Parameter Usage Frequency RMSE 29 implementations R² 19 implementations MAE 8 implementations
This structured process allows ML systems to adapt to varying conditions and deliver reliable insights for agricultural growth predictions.
Current Limitations of ML Models
Data Collection Issues
Machine learning has made strides in predicting plant growth, but collecting the necessary data remains a major challenge. According to the U.S. Department of Agriculture (USDA), only 27% of farms in the U.S. currently use precision agriculture practices. This leaves a significant gap in the data needed to improve these models.
Some of the key obstacles in data collection include:
- Inconsistent data formats, which make analysis difficult
- Limited rural internet bandwidth, preventing the transmission of large datasets
- Sensor calibration problems, which lead to inaccurate measurements
- A lack of standardized protocols, complicating the harmonization of data
These challenges are often caused by the variety of methods used to capture data and the difficulty of integrating information from multiple sensors .
User Experience Barriers
Even with sufficient data, user adoption remains a significant hurdle. Studies reveal that 50% of farmers are hesitant to invest in farm-management software, and 20% are concerned about data security. Trust also plays a big role in adoption. Surveys show that farmers place the greatest trust in agronomists, followed by neighboring farmers, sales reps, and finally online sources.
Weather and Season Effects
On top of technical and adoption challenges, environmental variability adds another layer of complexity. While machine learning models perform well under controlled conditions, unpredictable weather often leads to errors. For example, at an Australian wine farm, ML-powered disease prediction models helped reduce fungicide use by 30% without compromising grape quality. However, sudden changes in weather still limit the reliability of these models.
Research published in Nature Sustainability highlights the potential of ML in precision agriculture, showing it can reduce environmental impact by 35%. Yield prediction models generally achieve accuracy rates between 75% and 85%.
"Weather is a major determinant of crop yield... one of the biggest challenges is the inability to provide long-term weather forecasts." – Silke Migdall, managing director at VISTA remote sensing in geosciences
Changing climate patterns further complicate predictions. In regions like Rajshahi, seasonal patterns have become less predictable between 1981 and 2022. Heatwaves, for instance, are now occurring during the monsoon season, disrupting traditional farming cycles.
New ML Developments
3D Plant Monitoring
The latest advancements in 3D imaging have revolutionized how we track plant growth. Unlike older 2D methods, 3D monitoring systems offer a more detailed view by analyzing plant volume and identifying even the smallest structural changes. A recent study highlighted the power of this technology, reporting an 88.13% mean F1-measure and an 80.68% mean Intersection over Union (IoU) in detecting both new and existing plant organs using a spatiotemporal point cloud deep segmentation framework.
There are two primary approaches to 3D plant monitoring:
- Active Systems: These systems use controlled laser emissions to generate highly accurate 3D point clouds.
- Passive Systems: Relying on ambient light and multiple camera angles, these systems create detailed 3D reconstructions. While they are more cost-effective, they can produce noisier data.
Both methods allow for precise tracking of key growth indicators like canopy volume, branch angles, and fruit size. As these systems capture increasingly detailed data, efficient processing becomes a critical component of their success.
Distributed Learning Systems
Distributed learning has taken data analysis in agriculture to the next level. By scaling distribution 64 times, researchers have achieved a 99% reduction in simulation processing time and a 95% decrease in result aggregation time. This progress has been made possible by integrating big data frameworks, such as Apache Spark, with crop growth simulation models. Additionally, federated learning approaches ensure that massive datasets can be processed while maintaining data privacy.
Virtual Plant Models
Virtual plant models are reshaping how we simulate and optimize plant growth. Functional Structural Plant (FSP) models, often referred to as digital twins, represent a significant leap forward in growth simulation. These models incorporate a range of environmental factors to predict growth patterns and optimize growing conditions.
Recent implementations have achieved impressive results:
- Water Usage: A 44% reduction in irrigation needs.
- Fertilizer Efficiency: A 37% decrease in nitrogen fertilizer use through targeted application.
- Temperature Control: Models now maintain crop-specific temperatures, such as the optimal range of 50°F to 93°F for netted melons.
FSP models excel in replicating complex environmental interactions, including light interception, resource distribution, and plant-environment dynamics. They also help optimize pruning strategies and improve spray management efficiency. These virtual simulations are particularly beneficial in greenhouse settings, allowing growers to experiment with various management strategies without putting real crops at risk. This not only reduces potential losses but also ensures more efficient use of resources.
🚀 Ready to Reinvent Your Garden?
Join thousands of homeowners who have transformed their gardens using our AI design tool. Upload one photo to explore endless possibilities.
Get your AI garden designs →Looking Ahead
Summary Points
Machine learning is reshaping plant growth forecasting, with the AI agriculture market projected to jump from $1.7 billion in 2023 to $4.7 billion by 2028. This surge aligns with advancements in machine learning models and better integration methods.
One standout achievement is a deep convolutional neural network (DCNN) model that has reached an impressive 94% accuracy in identifying soybean stresses like bacterial and fungal diseases, nutrient deficiencies, and herbicide damage.
Modern monitoring systems are now pulling data from a variety of sources, including:
- Satellite imagery for large-scale analysis
- Drone surveillance for close-range monitoring
- Soil mapping to understand nutrient levels
- Real-time climate data for adapting to environmental changes
These tools are shifting agriculture from reactive problem-solving to proactive crop management. A key example comes from nitrogen management, where AI and remote sensing technologies have made a significant impact. Bilal Javed from Agriculture and Agri-Food Canada explains:
"Integrating AI with remote sensing technologies, such as drones and satellites, is transforming the way we manage nitrogen in agriculture. By providing real-time insights into machine learning algorithms, these innovations not only improve fertilizer efficiency but also enhance sustainability and optimize tuber yield."
These advancements are not just for large-scale farming - they’re also influencing practical tools for garden planning.
Garden Planning Applications
The same technologies driving agricultural innovation are now making their way into garden management. AIGardenPlanner is a prime example, leveraging machine learning to offer precise plant growth forecasting and tailored recommendations. Here's how it works:
- Climate-Based Analysis: The platform evaluates local weather trends to guide planting schedules.
- Soil Health Monitoring: Algorithms assess soil conditions and suggest specific fertilization strategies.
- Growth Rate Prediction: Machine learning models provide accurate estimates for plant growth and harvest timing.
Recent launches in ML-powered 3D vision systems are also enhancing garden planning by integrating data from multiple sources. These tools can detect plant stress long before symptoms appear, as seen in advancements like early detection of citrus greening disease. AI algorithms now make it possible to diagnose issues months ahead of visible signs, offering gardeners a proactive edge.
sbb-itb-4d6a8dd
Plantae Presents: Artificial Intelligence and Machine Learning in Plant Science
FAQs
How do machine learning models like Random Forest and XGBoost help predict and improve crop yields?
Machine learning models like Random Forest and XGBoost have become go-to tools for predicting crop yields. They excel at analyzing the complex relationships between factors like soil quality, weather conditions, and irrigation practices to provide reliable forecasts.
Take Random Forest, for instance. It's known for reducing prediction errors and delivering strong performance based on metrics like R² scores and Mean Squared Error (MSE). Meanwhile, XGBoost shines in more specific scenarios, producing highly accurate results when carefully fine-tuned to match the dataset. The choice between these models often hinges on the unique characteristics of the data and the specific objectives of the analysis.
By applying these sophisticated models, researchers and farmers can make smarter decisions, allocate resources more effectively, and boost agricultural output.
What challenges do farmers face when collecting data for machine learning in agriculture, and how can they overcome them?
Data Challenges in Agriculture
Farmers often encounter hurdles when it comes to gathering quality data for machine learning applications in agriculture. These challenges typically include incomplete or inconsistent data - whether it's sensor readings, weather data, or farm logs. On top of that, issues surrounding data privacy, ownership, and security can complicate the process of fully leveraging the information they collect.
So, how can farmers tackle these obstacles? One option is to use public datasets provided by government agencies or research institutions to fill in the gaps. Additionally, investing in reliable data management tools can help organize and safeguard sensitive information. Compliance with privacy regulations is also key to ensuring data security. Finally, adopting user-friendly software designed for agricultural data analysis can make it much simpler to transform raw data into useful insights that drive better decisions.
How is machine learning making home gardening smarter and more efficient?
🎨 Visualize Your Dream Garden Today!
Transform any outdoor space into a professional landscape design in minutes. Just upload a photo, choose your style, and let our AI do the rest.
Start your garden transformation now →How Machine Learning is Changing Home Gardening
Machine learning is reshaping the way we approach home gardening by introducing smarter, data-driven tools for plant care. With AI-powered systems, gardeners can now monitor key factors like soil moisture, sunlight levels, and temperature. These tools offer tailored recommendations for watering schedules, fertilization, and overall plant health, making it easier to manage resources effectively, conserve water, and grow healthier plants.
On top of that, AI technologies such as image recognition are a game-changer for plant disease management. They can detect signs of disease early, helping gardeners act quickly to protect their plants and maintain a flourishing garden. Thanks to these advancements, even hobby gardeners can adopt more efficient and resource-conscious gardening methods.